This article stems from an exploration of over 50 industry-specific AI use cases, guided by research insights from Google Gemini Deep Researcher, a custom-trained GPT model that I tailored for AI strategy and management consulting, and Perplexity AI. Over January 2025, I’ll share findings to demystify AI implementation, starting here with a focus on separating fact from fiction and avoiding colossal failures in AI projects.
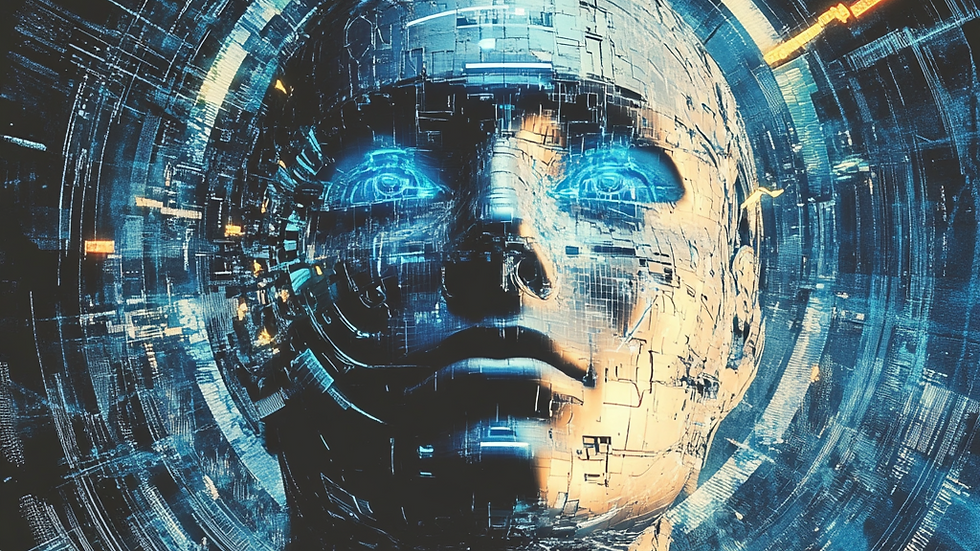
This article was originally published on LinkedIn by Severin Sorensen and has been approved for placement on Arete Coach. Scroll to continue reading or click here to read the original article.
A Personal Reflection on Early Tech Enthusiasm
The current AI buzz reminds me of the early 2000s when I worked in the physical security industry. Back then, vendors pitched video motion detection algorithms as the ultimate threat detection solution. However, it took nearly two decades for that vision to become viable.
Today’s AI feels similar. It promises transformative outcomes but also presents a journey fraught with challenges. While AI's potential is undeniable, leaders must approach it pragmatically, avoiding the trap of "demo pimps" peddling overpromised solutions. Real value emerges when AI implementation is grounded in thoughtful planning and clear objectives.
In my research, I’ve identified numerous promising AI use cases, which I’ll detail in future posts this month. What excites me most is the rapid evolution of AI models. Interacting with these tools increasingly feels like engaging with brilliant minds—challenging assumptions, sparking creativity, and elevating strategic thinking. The challenge now isn’t whether AI can deliver but how we can bridge the gap between its promise and meaningful results.
AI’s Grim Reality: 80% Failure Rate
The transformative promise of AI is undeniable. Yet, an analysis of 50+ use cases revealed a sobering statistic: over 80% of AI projects fail to reach completion—far higher than the failure rate of traditional IT projects (Ryseff, 2024). Why? AI, particularly agentic AI, demands fundamentally different approaches to design, deployment, and leadership.
Common Traps in AI Implementation
Unclear Objectives and Poor Project Management: Many AI projects start with enthusiasm but lack specific, measurable objectives. Weak project management leads to scope creep, misaligned priorities, and failure.
Insufficient Infrastructure and Data Governance: AI thrives on robust infrastructure and clean, well-governed data. Without these foundations, even the most ambitious projects are doomed.
Misunderstanding Agentic AI: Agentic AI systems aren’t static—they learn, evolve, and adapt. Misunderstanding this complexity leads to mismatched expectations and poorly designed systems.
Ignoring the Velocity Imperative: AI systems must adapt rapidly to changing business conditions. Without this agility, they quickly lose relevance and value.
Overlooking Architectural Humility: Overconfidence in AI's capabilities blinds organizations to its limitations. AI isn’t a magic wand; successful implementation requires humility, continuous learning, and system improvement.
The Wrong Leaders in Key Positions: One overlooked issue is leadership. Too often, organizations rely on IT leaders who excelled a decade ago but haven’t kept pace with AI’s demands. I’ve spoken with frustrated CEOs who recognize that outdated guidance from their teams hinders progress.
Building a Roadmap to Achievable AI
To harness AI’s transformative power, CEOs must adopt a disciplined, strategic approach. Here’s how:
Define Clear Objectives and Strengthen Project Management: Align AI initiatives with specific business goals. Invest in skilled project managers to translate technical capabilities into actionable plans.
Invest in Infrastructure and Data Governance: Build scalable, reliable infrastructure and implement rigorous data governance frameworks. Clean, accessible data is the lifeblood of AI success.
Deepen Understanding of Agentic AI: Educate leadership teams about agentic AI’s dynamic nature. Understanding its continuous learning process helps set realistic expectations and drives effective solutions.
Embrace the Velocity Imperative: Design systems that adapt quickly to changing market dynamics. Incorporate real-time data streams and algorithms that evolve alongside the business environment.
Promote Architectural Humility: Acknowledge AI’s limitations. Foster a culture of iterative development, continuous improvement, and realistic expectations.
Get the Right People in the Right Seats: As with finance or operations, having the wrong leader for AI strategy will hobble your organization. Be bold in recognizing when your current leadership isn’t fit for the modern challenges of AI—and act accordingly.
From Hopeium to Tangible Success
AI’s transformative power is real, but achieving results requires a shift from speculative optimism to disciplined execution. By addressing common pitfalls and aligning your organization with achievable strategies, you can turn potential failures into stepping stones to success.
Closing Thought: A CEO’s Role in AI Success
As a CEO, your role extends beyond championing innovation—you must also anchor it in reality. By steering clear of "hopeium" and focusing on strategic execution, you can guide your organization toward harnessing AI’s full potential.
This year, I’m dedicating my efforts to helping leaders navigate AI responsibly and effectively. Let’s turn AI’s promise into measurable outcomes—one pragmatic initiative at a time. Stay tuned for more insights in the weeks ahead.
References
Ryseff, J., Brandon De Bruhl, & Newberry, S. J. (2024, August 13). The Root Causes of Failure for Artificial Intelligence Projects and How They Can Succeed: Avoiding the Anti-Patterns of AI. Rand.org; RAND Corporation. https://www.rand.org/pubs/research_reports/RRA2680-1.html
Copyright © 2025 by Arete Coach LLC. All rights reserved.
Comments